Receiver Operating Characteristic analysis and the Cost – Benefit analysis in determination of the optimal cut-off point
Authors:
J. Vránová 1; J. Horák 2; K. Krátká 2; M. Hendrichová 2; K. Kovaříková 2
Authors place of work:
Univerzita Karlova v Praze, 3. lékařská fakulta, Ústav lékařské biofyziky a lékařské informatiky
1; Univerzita Karlova v Praze, 3. lékařská fakulta, I. interní klinika
2
Published in the journal:
Čas. Lék. čes. 2009; 148: 410-415
Category:
Přehledový článek
Summary
An overview of the use of Receiver Operating Characteristic (ROC) analysis within medicine is provided. A survey of the theory behind the analysis is offered together with a presentation on how to create a ROC curve and how to use Cost – Benefit analysis to determine the optimal cutoff point or threshold. The use of ROC analysis is exemplified in the “Cost – Benefit analysis” section of the paper. In these examples, it can be seen that the determination of the optimal cutoff point is mainly influenced by the prevalence and the severity of the disease, by the risks and adverse events of treatment or the diagnostic testing, by the overall costs of treating true and false positives (TP and FP), and by the risk of deficient or non-treatment of false negative (FN) cases.
Key words:
ROC analysis, ROC curve, Sensitivity, Specificity, Positive Predictive Value, Negative Predictive Value, Prevalence, Cost – Benefit analysis, Area under the Curve, Screening test, optimal cut point
Introduction
The ROC (Receiver Operating Characteristic) curve was first developed and used by American electrical and radar engineers during World War II for better detection of enemy objects on the battle field. Then it was employed in signal detection theory (1), (2). Later, ROC analysis was widely used in medical decision making, particularly in epidemiology, radiology and psychology (3). At present, ROC analysis was adopted in machine learning to evaluate and compare algorithms of neural networks and data mining methods (4), (5).
In medical decision making ROC analysis is increasingly used as a very powerful tool to determine the quality and discriminative ability of diagnostic or screening tests and of regression and discrimination models. It has been used in implementing new diagnostic tests, new drugs, and new therapeutic methods and to compare discriminative abilities among several different diagnostic tests in order to identify the preferred one. Today, Cost – Benefit analysis has became an inseparable part of ROC analysis.
Main characteristics of ROC analysis
ROC analysis is commonly used for two populations of patients (with and without specific disorder) because of the simple way it is defined and interpreted. It provides evaluation and graphical visualization of the behavior of classificators in the classification process.
When the results of a particular test in two populations of patients are considered, one with the disease, the other without the disease, a perfect separation between the two groups is rarely observed, indeed the distribution of the test results may overlap, as shown in Figures 2, 3 and 4. Therefore, after definition of a particular cut-off point or criterion value to discriminate between the two populations, these results are obtained:
- True Positive (TP) …cases with the disease correctly classified as positive
- True Negative (TN) … cases without the disease correctly classified as negative
- False Positive (FP) … cases without the disease, but classified as positive
- False Negative (FN) … cases with the disease incorrectly classified as negative
These four combinations can be entered into a special table (see Table 1), called a confusion matrix (6). In medical science and regression and discrimination analysis, this table is more commonly known as a classification table, because it shows the number of correctly and incorrectly classified cases.
From this table the following characteristics of ROC analysis can be defined:
- Sensitivity or TPR (True Positive Rate), defined as the probability that the test result will be positive when the disease is present, or as a ratio of cases correctly classified as diseased and all patients with the disease.
- Specificity or TNR (True Negative Rate), which is defined as the probability that the test result will be negative when the disease is not present, or as a ratio of cases correctly classified as normal (or healthy) and all healthy cases.
Next we defined the FPR (False Positive Rate) and FNR (False Negative Rate):
Note that: FPR = 1 – TNR and FNR = 1 – TPR.
The next important quantities of ROC analysis are predictive values for a diagnostic test:
PPV (Positive Predictive Value) defined as the probability that the disease is present when the test is positive, or as a ratio of true positive tests and all positive tests.
- NPV (Negative Predictive Value) defined as the probability that the disease is not present when the test is negative, or as a ratio of true negative tests and all negative tests.
Sensitivity and specificity are characteristics of the diagnostic test itself, but predictive values depend very strongly on the frequency of the disease in the population – the prevalence of the disease (also called pre-test or the prior probability that a subject has the disease before the diagnostic test is run). Using Bayes’ formula, adjusted values of PPV and NPV are calculated based on prevalence values as follows:,
Where P(D+) is the prevalence of the disease.
These predictive values (both, positive and negative) are the posterior probabilities of a subject having disease after the diagnostic test is conducted, and are of most interest to clinicians. So, a screening test is treated as a good test, if its results will increase the quality of the prognosis of the presence of the disease in comparison to the prediction based on the prevalence of the disease alone (7).
Another traditional characteristic of ROC curve analysis is:
- Accuracy of the screening test, which is defined as the ratio of the number of all correct diagnoses and the number in the total population.
Construction of a ROC curve (using Microsoft Excel spreadsheet)
Let’s imagine again, there are two populations – patients with a particular disease and a group of healthy normal individuals and there is a test which is positive if its value was above some defined cutoff value, and negative if below. The test is applied to each patient in each population in turn and a numeric result for each patient is determined. At first the data is sorted according to the test result value – largest value first. Now a table with four columns is created. The first column contains information about whether the patient has the disease or not. The second column gives the total number of patients with a test value greater than or equal to the test value for that row and the third and fourth columns contain the TPR (Sensitivity) and FPR (1 – Specificity) for each row. The ROC curve is constructed from the two values in the third and fourth columns. The ROC curve provides a visual comparison of the trade-offs between the true positive rate (Sensitivity on the vertical axis) and the false positive rate (1 – Specificity on the horizontal axis) of a diagnostic test for various cutoff values. The plot of the ROC curve together with optimal, strict and lenient thresholds respectively is shown in Figure 1. Now determine the last important quantity of ROC curve analysis – the Area under the ROC curve (referred to as AUC or AUROC), which is a measure of the accuracy of the test.
The Area under the ROC curve
The area under the ROC curve is non-parametric, and not significantly affected by the distributions of the underlying populations; therefore the non-normality of distributions is not a concern. In addition, the area under the ROC curve shows a clear similarity to the well-known Wilcoxon or Mann – Whitney U – test.
Possible values of the AUC, range from 0.5 (no diagnostic ability) to 1.0 (perfect diagnostic ability). A rough guide for classifying the accuracy of a diagnostic test is the traditional academic system (8):
- 0.50 – 0.60 … FAIL
- 0.60 – 0.70 … POOR
- 0.70 – 0.80 … FAIR
- 0.80 – 0.90 … GOOD
- 0.90 – 1.00 … EXCELLENT
If the area is 0.5 then the test is no better than flipping a coin.
Finding the Optimal Criterion Value
One of the most important tasks of ROC analysis is the determination of the optimal cutoff value. As seen in Figures 2, 3 and 4, if the position of the test threshold is varied, all other characteristics are also changed – TP, TN, FP, FN and consequently Sensitivity, Specificity, PPV and NPV.
As the test threshold is moved from left to right (as shown in Figures 2, 3 and 4, respectively) the corresponding point on the ROC curve (see Figure 1) also moves from left to right. The specific threshold moves from the “most strict” at the bottom left (point [0, 0] in Figure 1), gradually through the area of “strict”, “optimal” and “lenient” thresholds up to the “most lenient” at the top right (point [1, 1] in Figure 1). In the region of the strict decision threshold a larger amount of evidence is required in order to predict the patient’s disease. Strict thresholds (bottom inset) limit false positives at the cost of missing many affected individuals. Conversely, in the lenient region of thresholds, a smaller amount of evidence is required in order to predict the patient’s disease. Lenient thresholds (top inset) maximize discovery of affected individuals (almost all patients are classified as positive) at a cost of many false positives. The region of optimal thresholds – region closest to the upper-left corner, in which sensitivity and specificity are maximized – lies between these two regions. Where and under what conditions the cutoff point will be located for diagnosing a disease will be discussed later as examples in the section on “Cost – Benefit Analysis”.
In many scientific publications it is possible to see methods of cut point selection without any theoretical foundation or scientific justification. None of these methods considers the risks and benefits of over-treatment and under-treatment or the prevalence of the disease in clinical situations for which the diagnostic test is applicable (9). A review of studies found these methodology problems:
- an arbitrary point was selected, without any justification or explanation
- a point in the upper-left corner was selected, where Sensitivity and Specificity approach 100%
- the desired level of Sensitivity was predetermined and the value of Specificity was found from the curve
- the sum of Sensitivity and Specificity was maximized
- a point at which Sensitivity was equal to Specificity was chosen
Only ROC curve analysis involving Cost – Benefit analysis can estimate an optimal cut point in is essential for an approach to be truly scientific.
Cost – Benefit Analysis
Where cutoff point is placed for diagnosis of a disease is influenced by many criteria (10):
- Financial cost both direct and indirect of treating a disease (present or not), and of failing to treat a disease.
- Cost of further appropriate investigation.
- Discomfort to the patient caused by the treatment, or failure to treat.
- Mortality associated with treatment or non-treatment of the disease.
- Prevalence of the disease.
The optimal cut point of a diagnostic test is defined as the point at which the expected utility of a diagnostic test is maximized (10). This approach is based on an analysis of costs and benefits of the four possible outcomes of a diagnostic test: TP, TN, FP and FN. Once these costs are found, the average overall cost Cavg of performing a test is given by (10)
where, Cₒ is the overhead cost of actually doing the test, CTP is a cost associated with true positive, P(TP) is the proportion of TP’s in the population, and so on.
Metz (11) has shown that the optimal point on the ROC curve is the spot at which the slope R satisfies the following equation:,
where C … represents the net costs of treating nondiseased individuals
B … represents the net benefits of treating diseased individuals, and
P(D+) is the prevalence of disease.
The first term of the equation – the C/B ratio can be viewed in two ways – both negative and positive.
With the first perspective, “cost” is a negative outcome measure (monetary cost, adverse health risks, or a combination of the two). Therefore the C/B ratio can be expressed by the formula developed by Metz (11), Weinstein and Fineberg (12):
With the second perspective, when the positive point of view is considered, the C/B ratio may be viewed as “utility” (monetary savings, health benefits – better quality of life, a cure of the disorder, or a combination of both). Therefore the C/B ratio can be evaluated using the formula developed by Sox (13):.
The second term of the equation depends on the prevalence of the disease.
For a better understanding of the influence of both terms in the equation, consider these examples.
Prevalence
Consider a diagnostic test for detection of hepatitis B, which has a Sensitivity and Specificity equal to 0.99, and consider two populations (both with 10000 cases), one in Africa and China, where the prevalence of the disease is (14) 5 – 20% and the other in Europe, where the prevalence of hepatitis B is 0.1 – 1%. If you take into account the prevalence of the former being 20% and the later being 0.1% and enter these data into the confusion matrix – you get (see Table 2 and Table 3):
From these numbers both positive and negative predictive values can be calculated.
From the equation for the estimation of the optimal cut point, it can be seen that:
- For the first population (inhabitants of China and Africa, where the prevalence of the disease is very high, Table 2) the ratio
- Consequently, the cutoff point in the upper-right quadrant of the ROC curve plot should be chosen, where the line tangent to a point of the ROC curve has a relatively flatter slope. This point (Point A on Figure 1), also called the “lenient threshold”, minimized the number of false negatives, but also brought in many more false positives. But, as seen in Table 2, both values, PPV and NPV, are high enough, so that, if the disease is common, a positive test is likely to be a true positive. This fact minimized the number of false positives, so a cut point selection in the upper-right corner is exactly what is needed.
- On the other hand, for the second population (inhabitants of Europe, where the prevalence is low, Table 3) the ratio
- The line tangent to a point on the ROC curve has a steep slope and we have to choose a point from the lower-left corner of the ROC curve plot. The point from this part of the plot is called a “strict threshold” (Point B on Figure 1). This cutoff point yields fewer false positives, but at the expense of fewer true positives (false negatives increase). From the calculated values of PPV and NPV you can see that if the disease is rare, use of even a very specific test will be associated with many false positives (the value of PPV is very low). That is why the choice of a cutoff point in the region where in fact a small number of false positives are found, is the correct choice.
The kind of treatment and testing
Generally for diseases in which treatment or testing is toxic or dangerous to non-diseased patients, and in addition offers very little chance of a cure to diseased patients, the C/B ratio is large. Again this results in a steep slope – a “strict” cut point was selected, and both true positives and false positives are minimized. Conversely, when the cost of missing a diagnosis is great and treatment (even inappropriate treatment of a healthy person) issafe, a “lenient” cutoff point in the upper-right corner of the ROC curve plot should be used.
For a better understanding consider the following:
- Assume a particular disease – e.g. a brain tumor (this example was taken from (10)). If a positive test results, an operation on the brain of the patient is required (even if we know the operation is of little help to those with a cancer – i.e. many patients still die). If the test is negative, there is no intervention. Then the cost of false positives (FP) (a very dangerous operation on the open skull of a healthy person) is indeed far grater then the cost of true negatives (TN) (doing nothing), so CFP – CTN >> 1. The cost of false negatives (FN) (not doing the operation that doesn’t help much) is similar to true positives (TP) (doing a rather unhelpful operation), so CFN – CTP → 0 and
- and so a cutoff point in the lower-left quadrant should be chosen.
- Conversely, consider a patient with an appendicitis. With a positive test, an operation, which is safe, is required; so the cost of true positives (TP) is approximately the same as the cost of false positives (FP). The cost of true negatives (TN) is again zero (no intervention), but if you miss the diagnosis, the failure to diagnosis may be life threatening or even cause the patient’s death. So the cost of false negatives (FN) is enormous. So CFP – CTN → 0, CFN – CTP >> 1, and
- Therefore the cutoff point should be moved to the upper-right corner of the ROC curve plot.
Comparing two areas under the ROC curve
Very often, there is a need to compare different methods applied to the same data set and compare the ROC curves in order to determine which method is best. For this purpose Z – statistics should be used, defined by Hanley and McNeil (15) as follows:,
A1 and A2 are the two areas and SE1 and SE2 the corresponding standard errors and r is the quantity representing the correlation between the two areas due to working on the same set of data. If we applied two tests to different sets of cases then r = 0. Hanley and McNeil calculate standard error as:,
Where A is the area under the curve, nP and nN are the number of positive and negative (normal) values of the test respectively, and Q1 and Q2 are estimated by:
If Z is above a critical level, the null hypothesis H: “The two methods (areas) are the same” is rejectedand the alternative hypothesis HA: “The two areas are different” is accepted. It is important to point out that a non-significant difference between areas for two methods does not imply equivalence between the methods.
Summary
In our paper we present a short overview of ROC analysis together with Cost – Benefit analysis. We defined the main terms of ROC analysis – Sensitivity, Specificity, PPV, NPV and area under the ROC curve, and provided an explanation of the use of Cost – Benefit analysis in finding an optimal cutoff point. With respect to medical research, the main factors that influence our decisions are the prevalence of the disease, severity of the disease, toxicity of the diagnostic test or treatment, and the benefit of treatment for the patient.
Acknowledgments
This article was supported by Research Goal MSM 00 21620814 (“Prevention, diagnostics and therapy of diabetes mellitus, metabolic and endocrine damage of organism.”)
Abbreviations (Shortcuts)
ROC Receiver operating Characteristic
TP True Positive
TN True Negative
FP False Positive
FN False Negative
TPF True Positive Fraction
TNF True Negative Fraction
FPF False Positive Fraction
FNF False Negative Fraction
PPV Positive Predictive Value
NPV Negative Predictive Value
AUC (AUROC) Area Under the Curve
Ing. Jana Vránová, CSc.
Ústav lékařské biofyziky a lékařské informatiky
3. lékařská fakulta, Univerzita Karlova v Praze
Ruská 87,
100 42 Praha 10
Zdroje
1. Egan JP. Signal Detection Theory and ROC Analysis, Series in Cognition and Perception. New York: Academic Press 1975.
2. Swets JA, Dawes RM, Monahan J. Better Decision through Science. Scientific American 2000; 283: 82–87.
3. Beutel J, Kundel HL, van Metter RL. (eds) Handbook of Medical Imaging. Volume 1. Physics and Psychophysics. Bellingham, Washington: SPIE Press 2000.
4. Spackman KA. Signal detection theory: Valuable tools for evaluating inductive learning. In: Proceedings of the Sixth International Workshop on Machine Learning. San Mateo, CA: Morgan Kaufman 1989; 160–163.
5. Skalská H. Statistika a technologie data mining. Hradec Králové: 2000; habilitační práce.
6. Zavadil Z. Způsoby vyhodnocování kvality separace dvou a více množin, metody vizualizace výsledků, rešeršní práce. ČVUT FJFI, Katedra matematiky 2004.
7. Zvárová J, Hanzlíček P, Hejl J, Jirkovec Z, Pikhart H, Přibík V, Smitková V, Zvára K. Základy informatiky pro biomedicínu a zdravotnictví [online]. EuroMISE Centrum 2006, [cit. 2008-11-13], http://www.euromise.cz/education/textbooks/ biomedicinska_informatika.html.
8. Tape TG. Interpreting Diagnostic Tests [online], University of Nebraska Medical Center, [cit. 2008-11-13], http://gim.unmc. edu/dxtests/ROC3.htm.
9. Cantor SB, Sun CC, Tortolero-Luna G, Richards-Kortum, Follen M. A Comaprison of C/B Ratious from Studies Using Receiver Operating Characetrsistic Curve Analysis. J Clin Epidemiology 1999; 52: 885–892.
10. The Magnificent ROC [online], [cit. 2008-11-13], http://www. anaesthetist.com/index.htm.
11. Metz CE. Basic Principles of ROC Analysis. Semin Nucl Med 1978; 8: 283–298.
12. Weinstein MC, Fineberg HV. Clinical Decision Analysis. Philadelphia: W. B. Saunders 1980.
13. Sox HC, Blatt MA, Higgins MC, Marton KI. Medical Decision Making. Boston: Butterworths 1988.
14. Adam Z, Ševčík P, Vorlíček J, Mistrík M. Kostní nádorová choroba. Praha: Grada Publishing, a.s. 2005.
15. Hanley JA, McNeil BJ. A Method of Comparing the Areas under the Receiver Operating Curves Derived from the Same Cases. Radiology 1983; 148: 839–843.
Štítky
Adiktologie Alergologie a imunologie Anesteziologie a resuscitace Angiologie Audiologie a foniatrie Biochemie Dermatologie Dětská dermatologie Dětská gastroenterologie Dětská gynekologie Dětská chirurgie Dětská kardiologie Dětská nefrologie Dětská neurologie Dětská onkologie Dětská otorinolaryngologie Dětská pneumologie Dětská psychiatrie Dětská radiologie Dětská revmatologie Dětská urologie Diabetologie Endokrinologie Farmacie Farmakologie Fyzioterapie Gastroenterologie a hepatologie Genetika Geriatrie a gerontologie Gynekologie a porodnictví Hematologie a transfuzní lékařství Hygiena a epidemiologie Hyperbarická medicína Chirurgie cévní Chirurgie hrudní Chirurgie plastická Chirurgie všeobecná Infekční lékařství Intenzivní medicína Kardiochirurgie Kardiologie Logopedie Mikrobiologie Nefrologie Neonatologie Neurochirurgie Neurologie Nukleární medicína Nutriční terapeut Obezitologie Oftalmologie Onkologie Ortodoncie Ortopedie Otorinolaryngologie Patologie Pediatrie Pneumologie a ftizeologie Popáleninová medicína Posudkové lékařství Praktické lékařství pro děti a dorost Protetika Psychologie Radiodiagnostika Radioterapie Rehabilitační a fyzikální medicína Reprodukční medicína Revmatologie Sestra Sexuologie Soudní lékařství Stomatologie Tělovýchovné lékařství Toxikologie Traumatologie Urgentní medicína Urologie Laboratoř Domácí péče Foniatrie Algeziologie Zdravotnictví Dentální hygienistka Student medicínyČlánek vyšel v časopise
Časopis lékařů českých
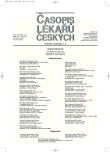
- Metamizol jako analgetikum první volby: kdy, pro koho, jak a proč?
- Není statin jako statin aneb praktický přehled rozdílů jednotlivých molekul
Nejčtenější v tomto čísle
- Receiver Operating Characteristic analysis and the Cost – Benefit analysis in determination of the optimal cut-off point
- Subacute thyroiditis confused with dental problem
- Fractional exhaled nitric oxide and its correlation with bioptic results in chronic cough patients
- Zoonózy – nákazy s přírodní ohniskovostí