Differences in sleep patterns among healthy sleepers and patients after stroke
Sleep deprivation, whether from disorder or lifestyle, whether acute or chronic, poses a significant risk in daytime cognitive performance, excessive somnolence, impaired attention or decreased level of motor abilities. Ischemic stroke resulting in cerebral lesions is a well-known acute disorder that leaves affected patients strongly vulnerable to sleep disturbances that often lead to the above-mentioned cognitive and attentional impairments. In this paper, we analyzed and compared sleep patterns of healthy sleepers and patients after stroke. To overcome the well-known limits of the standardized sleep scoring into several discrete sleep stages we employed the recently proposed probabilistic sleep model that represents the sleep process as a continuum in terms of a set of probability curves. The probability curves were considered to represent a form of functional data, and microstructure along with time dynamics of the curves were studied using functional principal components analysis and clustering. Although our study represents a preliminary attempt to separate the two groups of subjects, we were able to identify several physiologically separate sleep patterns and we also identified sleep microstate patterns being a potential source allowing the discrimination of healthy subjects and stroke patients.
Keywords:
sleep patterns, stroke, probabilistic sleep model, functional principal components analysis
Authors:
Bui Thi Mai Phuong; Roman Rosipal
Authors place of work:
Slovak Academy of Sciences, Bratislava, Slovak Republic
; Department of Theoretical Methods, Institute of Measurement Science
Published in the journal:
Lékař a technika - Clinician and Technology No. 4, 2014, 44, 15-20
Category:
Původní práce
Summary
Sleep deprivation, whether from disorder or lifestyle, whether acute or chronic, poses a significant risk in daytime cognitive performance, excessive somnolence, impaired attention or decreased level of motor abilities. Ischemic stroke resulting in cerebral lesions is a well-known acute disorder that leaves affected patients strongly vulnerable to sleep disturbances that often lead to the above-mentioned cognitive and attentional impairments. In this paper, we analyzed and compared sleep patterns of healthy sleepers and patients after stroke. To overcome the well-known limits of the standardized sleep scoring into several discrete sleep stages we employed the recently proposed probabilistic sleep model that represents the sleep process as a continuum in terms of a set of probability curves. The probability curves were considered to represent a form of functional data, and microstructure along with time dynamics of the curves were studied using functional principal components analysis and clustering. Although our study represents a preliminary attempt to separate the two groups of subjects, we were able to identify several physiologically separate sleep patterns and we also identified sleep microstate patterns being a potential source allowing the discrimination of healthy subjects and stroke patients.
Keywords:
sleep patterns, stroke, probabilistic sleep model, functional principal components analysis
Introduction
Sleep is a time-dynamic process coming in diverse forms. Polysomnography (PSG) is the standard diag-nostic procedure for capturing the structure of sleep. It consists of a battery of diagnostic tests monitoring the subject's cerebral and cardial activity, eye movement, muscle tension, breath rate, and other physiological and neurophysiological variables, throughout the whole night. The key component of PSG is the recording of brain electrical activity on scalp, the electroencep-halography (EEG). A hypnogram, a form of PSG, represents the graph of clinically well-defined sleep stages as a function of time. It is usually obtained by visual scoring of EEG, electrooculogram (EOG) and electromyogram (EMG) recordings. Cycles of rapid eye movement sleep (REM) and non-rem (NREM) stages are the major components of the sleep process. According to the scoring protocol of the American Academy of Sleep Medicine (AASM), NREM sleep can be further classified into stage 1, 2, and 3, which includes previously considered stage 4 of the Rechtschaffen and Kales (RK) scoring protocol into stage 3 [1, 2]. This stage is called slow wave sleep (SWS) and represents the deepest sleep.
Strokes are caused by an interruption of blood flow to the brain. If the condition persists for more than a few seconds, it results in the loss of consciousness and leads to brain ischemia, consequently resulting in irreversible brain disorders and brain tissue damage. Sleep disorders may be direct functional consequences of a stroke. For example, obstructive sleep apnea is the major sleep disorder associated with an ischemic stroke [3]. On the other hand, sleep disruption, partial or full sleep deprivation is ubiquitous following hemispheric strokes. Characterizing the sleep structure of brain-damaged patients is a challenging task, since the condition of patients often does not allow for any measurement to take place. Targeted experiments with a proper monitoring and evaluation of sleep are scarce; studies in this area are therefore limited. We highlight but a recent study by Jiang et al. [4] who found polysomnographic abnormalities in patients with a specific cognitive impairment. The results hint at the potential of this line of research.
This paper summarizes initial work within the national project titled "Effects of sleep disturbances on day-time neurocognitive performance in patients with stroke". The project aims to answer the following three questions: a) Are there differences in sleep patterns between healthy sleepers and stroke patients? b) How does sleep affect the subjects' daytime neurocognitive performance? c) Is the sleep-performance relationship different in healthy subjects and patients with stroke? Here, we focus on and provide a discussion of the first problem. In particular, we carry out a statistical analysis and evaluation of sleep EEG recordings.
The paper is organized as follows. First, we provide technical details about the dataset in question and about the preprocessing steps taken. Next, we note that the raw EEG time series is in itself cumbersome to work with and on that account we seek a more workable transcription of sleep. This leads us to the probabilistic sleep model (PSM). The PSM produces a new sleep representation in the form of posterior probability curves representing sleep microstructure defined by a finite set of sleep microstates. Subsequently, we treat the posteriors as functional data and tackle the problem using tools of functional data analysis. Finally, we give the results of applying this procedure to our dataset.
Method
Data specification and preprocessing
In total, 99 whole-night EEG recordings were analyzed, 34 of which correspond to stroke patients. These had been collected in accordance with the objectives of the project, not later than 7, and in most cases within 5 days after the occurrence of stroke. A sample of recordings of healthy sleepers taken from the SIESTA database [5] was used as the control group. The database consists of full PSG recordings of two consecutive nights for 175 healthy adults of varying ages. However, as one would expect, stroke patients are mostly elderly people. Since age is one of the major factors of sleep architecture, only healthy subjects over 60 were included in our study to avoid age related bias in the analysis. The first-night recording was taken, since adaptation to the clinical environment may influence subsequent measurements. All subjects in the control group had a Pittsburgh Sleep Quality Index [6] of at most 5.
A brief quantitative characterization of the dataset is summarized in Table 1.
The RK hypnograms used for sleep marker computation had been obtained by manual scoring for stroke patients and via an automatic RK classifier, Somnolyzer 24x7 [7], in the case of healthy subjects.
Though it would be interesting to look at other PSG components as well, we left it as a future research question and used EEG data only. Primarily, the C3 EEG channel referenced to M2 was considered. Noise-infected segments were replaced by corresponding C4-M1 channel segments, if artifact-free, otherwise the problematic time intervals were ignored. All data were down-sampled to 100 Hz.
Probabilistic sleep model
The PSM is a novel sleep model proposed and validated by Lewandowski et al. [8]. For the complete description of PSM, we refer the reader to [8]. Subsequent sleep process related validation of the model was carried out in [9]. Below we provide a brief summary of the model.
Based on the specific PSG patterns produced, various forms of sleep may be identified. The traditional RK classification [2] distinguishes between phases involving REM and NREM sleep. Phases of NREM sleep are further classified according to depth as S1, S2, S3 or S4, in order from lighter to deeper sleep. S3 and S4 are considered as SWS.
The classical representation of sleep, the hypnogram, is then derived by dividing a PSG recording into 30 s long epochs and assigning each epoch to one of the five or six classes mentioned above. The scoring is usually performed by an attending specialist according to the RK rules [2].
The RK model has been criticized for dependence on inherently subjective judgments involved in sleep stage classification. Also, it is at best a crude description of sleep, both in terms of time resolution and the simplistic classification into a small number of fixed stages. The PSM aims to address these issues by introducing two main changes. First, the considered time window of an epoch is at the scale of a few seconds (in this work 3 s), constituting a compromise between a more fine-grained partition and the ability to capture low-frequency EEG waves with reasonable reliability. Second, and more importantly, it explains sleep in terms of a larger number of sleep states, which we denote microstates, and transitions between them. Instead of a class label, the PSM assigns each time window a vector of non-negative values summing to one. The components of the vector correspond to the considered microstates and may be interpreted as weights or probabilities that the subject was in the respective microstate during the considered time epoch. Thus, the PSM is able to capture a much wider range of sleep forms.
Plotting probabilities that apply to a given microstate against time produces a posterior curve. A complete PSM transcription of sleep comprises a set of posterior curves, one for each microstate.
The PSM construction
The PSM relies on a sample of PSG recordings to identify and learn the typical characteristics of the hypothesized ensemble of sleep microstates. It follows that the behavior of a PSM, and consequently all posteriors derived from it, depend on the training dataset. In particular, the training dataset and the posteriors generated by the PSM are not statistically independent. We will return to this issue in the later section. Below we give an overview of the training procedure we used in the presented work.
- Input: A training dataset consisting of m whole-night EEG recordings.
- Partition each EEG time series into disjoint 3 s epochs. Obtain {x(i) | i = 1, ..., n}, where n is the total number of epochs in all m recordings. At this stage, the time ordering of epochs is ignored. Provided the EEG is sampled at 100 Hz, x(i) is a vector of 300 data points corresponding to the i-th epoch.
- Fit an autoregressive model (AR) of order 10 to each epoch. Symbolically,
- Fit a Gaussian mixture model (GMM) to the observations. Here, a parametric model is introduced to explain the distribution of the set of AR coefficients {y(i) | i = 1, …, n}. Each observation is assumed to have been generated by one out of k microstates. Each microstate occurs with a fixed probability πj and is assumed to generate AR vectors from a Gaussian distribution. Formally, the assumed density is given by
- where πj , μ(j) and Σ(j) are unknown parameters which are estimated from the training data using the principle of maximum likelihood estimate (MLE). In the absence of a closed-form expression for the MLE, estimates are derived using the expectation-maximization (EM) algorithm, as is common practice in a GMM context.
- Output: The fitted Gaussian mixture model. The optimal values of parameters have a natural interpretation. Variables μ(j) , Σ(j) represent centers and covariance matrices of the identified clusters and πj indicates how common a microstate is.
Note that a variant of the PSM can exploit additional training information in the form of RK and/or spindle labels to assign RK meaning to microstates. The RK-based model can combine microstate posteriors to produce a set of RK posteriors, which may be conceived of as a continuous counterpart of the hypnogram. This is in contrast to the version of the PSM presented here, which is a pure model in the sense that it aims to define sleep microstates based solely on the data, unaffected by any a priori RK based scoring.
The PSM in action
A successfully trained PSM can be used for converting sleep EEG data into a set of posterior probabilities. The following mechanism is applied in this step.
- Input: An EEG signal from a whole-night sleep recording.
- Partition the signal into 3s time frames and fit an AR(10) model to each. Thus obtain nt vectors of AR coefficients {y(i) | i=1, ... , nt} representing EEG data.
- For i = 1, ..., nt, evaluate the responsibility of each microstate for the i-th data segment. The responsibility γ(i)j represents the probability that microstate j generated y(i), conditional on the value of y(i)
- The posterior curve for microstate j is given by
- Output: Microstate posterior curves.
It should be noted that while RK stages have a well-known, clinically established physiological meaning, the major physiological attribute that characterizes a microstate is the corresponding 10-dimensional Gaussian itself. There is a plausible way of assigning meaning to a microstate via the center of the Gaussian, a typical representative of the microstate. From the vector of AR coefficients, the microstate-characteristic frequency spectrum can be recovered and easily read [8, 9].
Functional principal component analysis
For the purposes of further analysis, the representation of sleep in terms of a set of posterior curves is still complex. We therefore sought a form of data reduction. At the same time, it was desirable that the transformation retains time-related properties of posterior curves, so that the information on the variation of sleep in time is preserved.
One such technique, and a common first step in continuous-curve-data mining, is the functional principal component analysis (fPCA). As the name suggests, the method represents an alternative to the standard statistical PCA technique of extracting directions of maximal variance, designed for functional data. In fPCA the original posterior curves are represented in a new basis providing a lower-dimensional representation. Computationally, the process involves finding dominant eigenvalues and eigenfunctions of the variance-covariance function estimated from the posterior curves. For a detailed treatment of fPCA and related methods we refer the reader to [10]. We used the PACE implementation of fPCA [11].
Sleep posterior curves clustering
In the following, we detail the steps taken in applying functional data analysis techniques to our dataset. We trained an RK-free PSM using all 99 subjects as the training set and computed posteriors for each subject. On the one hand, by fitting the model to all available data, the subjects were treated sym-metrically and we avoided the uncertainty connected with either random or criterion-based training set selection. On the other hand, converting recordings that had already been used for model fitting violates the inter-subject statistical independence of the resulting posteriors. Nevertheless, this led to a faithful and rich representation of sleep. Effectively, we sacrificed independence for greater explanatory power, which is justifiable in an exploratory context used here.
The model was set to comprise 15 Gaussian mixture components. The number was chosen empirically as a compromise between reasonable adaptive power and stability of the model. Concerns over the model's instability arise from the PSM's definition via maximum likelihood, which includes the EM algorithm that tends to converge to local extrema.
Next, PSM-based posteriors were derived and processed using positive spline smoothing. Each out of 99 available polysomnograms was thus represented by 15 smoothed microstate posterior curves. For each microstate, fPCA was performed on the respective 99 curves leading to a reduction to lower-dimensional vectors of scores.
Seeking structure in the data, the vectors were subsequently clustered using the 2-means algorithm. The procedure was performed repeatedly, with cluster centers initialized as two randomly selected data points in each run. Although the algorithm did not always converge to the same state, the results generally differed by fewer than three subjects. In Figure 1, we consider the clustering given by the 2-means method with initial cluster centers chosen as the mean vector across stroke subjects and the mean across the control group. The fraction of variance explained by fPCA was set to 85%, but we observed that varying the number of principal components had a minor effect on the resulting clusters. The assumption underlying this procedure is that if there is no significant difference in sleep architecture of the two populations, then the clusters will be roughly independent of the healthy-stroke partition.
Results and Discussion
Results for each sleep microstate are summarized in Figure 1. Taking into account the different lengths of recordings, the value of a representative posterior in a given time point is computed as the mean across available posterior values in that time point. The physiological interpretation of individual microstates can be inferred from their frequency spectra as well as from posteriors. As a rule of thumb, deeper sleep occurs later in the night and is associated with lower-frequency waves.
It can be observed that microstates 2 and 10 are more representative of patients with stroke. We find microstate 2 very interesting, as the cluster posteriors follow the two groups fairly closely from about the middle of the night towards the morning. In our future investigations, the microstate will be explored in greater depth, for example by analyzing the second half of the night separately.
Microstates 3 and 12 capture the specificity of sleep of a small subset of healthy subjects. As can be noted from high probability values, the microstates explain a considerable component of these subjects' sleep. In the next step, a hierarchical clustering method should be used to separate the specific subjects. Alternatively, we could increase the number of clusters.
Microstate 4 describes an aspect of healthy sleep as well, but corresponds to a rather different physiological state and involves a greater portion of the population. Due to the fact that one of the clusters includes virtually all stroke patients, there is a fairly close fit between this cluster's and stroke patients' posterior curves.
Based on its spectral and temporal characteristics, microstate 9 could be seen as a close-to-wake state, highly typical of healthy individuals and virtually unachievable by patients after stroke. Especially values of posteriors early in the night differ markedly between the two groups. Microstate 15 is also a quasi-wake state related mainly to healthy subjects, but in contrast, it seems capturing differences between the groups later in the night. Apart from the already mentioned microstates 9 and 15, sleep microstates 1 and 13 also seem to be closely linked to wake. In neither of these four microstates does the stroke mean posterior dominate the posterior of healthy subjects. On the contrary, stroke patients are more dominant in connection with microstates 2, 8 and 10, which correspond to SWS.
Conclusions
In this paper, we analyzed and compared sleep patterns of healthy sleepers and subjects with stroke. We used the probabilistic sleep model to obtain a tractable but exhaustive representation of sleep and proceeded with the analysis using an approach that combined functional principal component analysis with 2-means clustering.
Our search did lead to interesting findings. We detected notable differences in sleep microstructure of the studied populations. These differences were also reflected in macro-level sleep descriptors such as the subject's overall inclination towards wake or SWS. We recognized considerable within-group variation of the sleep process, especially in the case of healthy subjects. No obvious pathologies in the sleep of stroke patients were detected.
In addition to the obtained results, we see the contribution of the paper in demonstrating the descriptive power of the PSM, either as a continuous alternative to the RK hypnogram, or as a standalone detailed transcription of sleep. Besides, we hinted at the potential of further research in this area of sleep neurophysiology, and at the multitude of ways to tackle problems involving neurophysiological signals.
Still, our study leaves open a number of questions. There is still much to be learned from our dataset and we will follow the presented work with a more in-depth investigation. A project is under way to ascertain whether the differences in sleep architecture affect stroke patients' daytime neurocognitive performance. Also, it would be interesting to study a large enough stroke sample that would permit a classification based on the type, position and severity of stroke, and a whole new realm of possibilities would open up by incorporating other polysomnographic channels, most notably the electromyogram and electrooculogram.
Acknowledgement
We would like to thank personnel at the First Department of Neurology, Faculty of Medicine, Comenius University in Bratislava for providing us sleep EEG data of stroke patients and for thorough clinical consulting. This work was supported by the MZ 2013/56-SAV-6, APVV-0668-12, VEGA 2/0043/13 and VEGA 1/0503/13 research grants.
Bui Thi Mai Phuong
Department of Theoretical Methods
Institute of Measurement Science
Slovak Academy of Sciences
Dúbravská cesta 9, 84104 Bratislava
E-mail: mariana.phuong@gmail.com
Phone: +421 944 124 247
Zdroje
[1] Iber, C., Ancoli-Israel, S., Chesson, A. & Quan, S. The AASM Manual for the Scoring of Sleep and Associated Events: Rules, Terminology and Technical Specifications. American Academy of Sleep Medicine, 2007.
[2] Rechtschaffen, A. & Kales, A. A manual of standardized terminology, techniques and scoring system for sleep stages of human subjects. 1968.
[3] Kapen, S., Park, A., Goldberg, J. & Whynter, J. The incidence and severity of obstructive sleep apnea in ischemic cerebrovascular disease. Neurology, 1991, vol. 41, no. 125.
[4] Jiang, B., Ding, C., Yao, G., Yao, C., Zhang, Y., Ge, J., Qiu, E., Elia, M. & Ferri, R. Polysomnographic abnormalities in patients with vascular cognitive impairment - no dementia. Sleep Medicine, 2013, vol. 14, p. 1071–1075.
[5] Klösch, G., Kemp, B., Penzel, T., Schlögl, A., Rappelsberger, P., Trenker, E., et al. The SIESTA project polygraphic and clinical database. Engineering in Medicine and Biology Magazine, 2001, vol. 20, p. 51–57.
[6] Buysse, D. J., Reynolds III, C. F., Monk, T. H., Berman, S. R. & Kupfer, D. J. The Pittsburgh Sleep Quality Index: a new instrument for psychiatric practice and research. Psychiatry Research, 1989, vol. 28, p. 193–213.
[7] Anderer, P., Gruber, G., Parapatics, S., Woertz, M., Miazhynskaia, T., Klösch, G., et al. An E-health solution for automatic sleep classification according to Rechtschaffen and Kales: validation study of the Somnolyzer 24x7 utilizing the SIESTA database. Neuropsychobiology, 2005, vol. 51, p. 115–133.
[8] Lewandowski, A., Rosipal, R. & Dorffner, G. Extracting more information from EEG recordings for a better description of sleep. Computer Methods and Programs in Biomedicine, 2012, vol. 108, p. 961–972.
[9] Rosipal, R., Lewandowski, A. & Dorffner, G. In search of objective components for sleep quality indexing in normal sleep. Biological Psychology, 2013, vol. 94, p. 210–220.
[10] Ramsay, J.O. Functional data analysis. Wiley Online Library, 2006.
[11] Yao, F., Müller, H.G., Clifford, A.J., Dueker, S.R., Follett, J., Lin, Y., et al. Shrinkage estimation for functional principal component scores with application to the population kinetics of plasma folate. Biometrics, 2003, vol. 59, p. 676–685.
Štítky
BiomedicínaČlánek vyšel v časopise
Lékař a technika
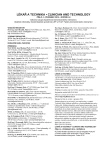
2014 Číslo 4
Nejčtenější v tomto čísle
- REAL-TIME visualization of multichannel ECG signals using the parallel CPU threads
- Differences in sleep patterns among healthy sleepers and patients after stroke
- Pulmonary fluid accumulation and its influence on the Impedance Cardiogram: CompariSON Between a Clinical Trial AND FEM Simulations
- EFFECT OF THE PLACEMENT OF THE INERTIAL SENSOR ON THE HUMAN MOTION DETECTION
Zvyšte si kvalifikaci online z pohodlí domova
Kardiologické projevy hypereozinofilií
nový kurzVšechny kurzy