EEG MICROSTATES ANALYSIS IN PATIENTS WITH EPILEPSY
Authors:
Vaclava Piorecka 1,2; Marek Piorecký 1,2; Jan Strobl 1,2; Marie Nezbedova 1; Hana Schaabova 1; Vladimir Krajca 1
Published in the journal:
Lékař a technika - Clinician and Technology No. 3, 2018, 48, 96-102
Category:
Original research
Summary
Analysis of microstates in electroencephalographic recordings (EEG) is a promising topographical method that is currently being studied for the diagnosis of neuropsychiatric diseases. The aim of our study is to describe the feasibility of using the microstate analysis of EEG for examination of the epileptic patients. The EEG recordings were measured on patients with epilepsy and on control subjects (with no epileptic pathology). We calculated the global field power (GFP) curve to extract microstates from the EEG recordings. We took local maxima (peaks) of GFP curve to create amplitude topographic maps. Four microstates can be found or are proven to be found in physiological activity, sleeping, and in some pathological activity (schizophrenia). Our goal is to find out if the same microstates also occur in patients with epilepsy. Our assumption is that, if all four identical microstates are present in EEG with epileptic activity, the parameters of these microstates should differ between the EEG of a healthy individual and a person suffering from epilepsy. We observed that the microstate 1 seems to have a higher occurrence for the non-epileptic controls than the patients with epilepsy. The duration of the microstate 4 seems to be higher in the epileptic patients than the non-epileptic controls. We have found that there is a significant difference in the duration, occurrence and contribution of the amplitude topographic maps between the non-epileptic controls and the patients with epilepsy.
Keywords:
EEG, Microstates, GFP, Epileptic activity
Introduction
The electroencephalographic recordings (EEG) are useful for analyzing brain activity and they are capable of detecting pathological conditions such as epilepsy. One of the methods of EEG analysis is the calculation of microstates and the description of their parameters that are supposed to describe specific brain states. A microstate analysis is a method that describes the EEG through maps of electrical potentials that are caused by the electrical activity of the brain. The elec-trical potential maps are obtained by scanning the surface of the skull using a multi-channel array of electrodes. The microstate is defined as a short time period during which the EEG topography remains quasi-stable. This means that global topography is solid, but the strength may vary and invert the polarity. The microstates remain stable for a certain period of time 60–120 ms. [1, 2]
In the spontaneous EEG, four standard microstate classes were distinguished in [1], these classes are being denoted by letters A, B, C, D, where each class has a different map orientation. The map of the class A has a left posterior–right anterior orientation, for the class B the map has a right posterior–left anterior orientation, the class C has an anterior–posterior orientation and the class D has a central maximum (midline frontal topo-graphy) [3]. Classes are often described by parameters such as duration, frequency, amplitude, topographic shape, etc. Four classes of microstates parameters were investigated in various stages of evolution in the study [4]. We aim to show that the microstate analysis is feasible for the epileptic EEG recordings and not only for physiological state or other neuropsychiatric diseases found in the literature.
With advances and developments in neuro-physio-logical techniques, a better idea of brain functioning is gained and the principle of distortion of this brain function in the disease is being understood. Also, the microstate analysis is often researched and used to study and evaluate the overall function of the brain in health and in disease. This method is relatively quick, available and inexpensive. Patients are examined with open or closed eyes or with different visual or auditory stimuli. The mentioned studies show that all 4 microstates classes are present, and all four microstates are needed to form a visual and verbal thought. Visual and verbal conditions were predominantly associated with micro-states A and B. [3, 5]
Currently, the microstate analysis is often used to investigate and diagnose neuropsychiatric diseases. With these diseases, the specific parameters describing individual microstate may change significantly. Some classes may occur more frequently or vice versa, they may change in the duration and they show different specific transitions, etc. [1]. The microstates exami-nation in patients with schizophrenia [1, 6] shows differences in microstates parameters with a comparison to healthy subjects. The average time of occurrence of two microstates (B and D) was significantly reduced, whereas microstates A and C appeared more frequently. In the patients with schizophrenia, the microstate B was significantly altered. The control subjects showed a specific transition of microstates A → C → D → A and the schizophrenic patients showed a specific transi-tion of microstates A → D. [1, 6]
In the study [7] of dementia, a significantly shorter total average duration of microstates was found. In patients with panic disorder [8], the results indicated a prolongation of the microstate A and the frequency of occurrence in the microstate C was lower. Also, microstates have been investigated in diseases such as Alzheimer’s disease [7], depression [9], Tourette’s syndrome [9], etc. The investigation of the disease by microstates analysis offers a new approach to detecting and monitoring the severity of the disease through objective neurophysiological biomarkers. [9]
To the best of our knowledge, no microstate analysis applied to epilepsy has been published yet.
The epilepsy is a neurological disease of the brain characterized by recurrent seizures. The epileptic sei-zures are the result of the transient and unexpected electrical disturbances of the brain. This disease affects millions of people around the world. The most common diagnostic method for detecting epilepsy is the EEG signal analysis. The interconnection of various diagnostic methods (for example magnetoencepha-lography, magnetic resonance and EEG) for studying epilepsy serves to a deeper understanding of the processes that control and regulate seizure activity. [10, 11]
The functional magnetic resonance (fMRI) has an excellent spatial resolution. It allows the localization of areas where the level of neuronal activity changes during the experimental period. The conventional analysis of EEG-fMRI data is based on the visual identification of the interictal epileptiform discharges (IEDs) on scalp EEG. [12]
This paper studies the feasibility of microstates analysis in EEG recordings of patients with epileptic activity. It starts with verification of the studies found in literature—finding the microstates and their parameters in non-epileptic controls and then analyzing the EEG recordings with epileptic activity in the same way to confirm the feasibility. The feasibility study includes testing of the microstate analysis on a small dataset and with a view to verifying the prospects of using micro-states analysis for the recordings with epileptic activity.
This paper shows that it is also possible to distinguish between epileptic and non-epileptic EEG recordings using the microstate analysis, therefore an analysis of parameters is made to find out if some parameters change in a different way than in non-epileptic controls. The main goal of this study is to support the decision of the physicians in the way of classifying epilepsy and not only in the way of the detection of the epileptic seizures [13].
Data
Our study includes 10 controls and 6 subjects suffering from epilepsy. Tested subjects were men and women aged 19–58 years. The study protocol has been approved by the Hospital Na Bulovce ethical committee.
The EEG data are 19-channel-ambulatory EEG recordings measured in the Hospital Na Bulovce in standardized conditions using the 10-20 system EEG cap with reference electrodes on earlobes. The record-ings were measured on the Brain-Quick (Micromed S.p.A.) digital system. The lengths of recordings ranged from 15 to 54 minutes. The sampling frequency of EEG recordings of the patients with epilepsy is 128 Hz. Non-epileptic control EEG recordings were measured with a sampling frequency of 256 Hz and down-sampled to 128 Hz. The sensitivity was 100 μV per 10 mm. The electrode impedances were not higher than 5 kΩ. The data were filtered with a bandpass filter 0.5–30.0 Hz.
Microstates studies usually used bandpass filters with the upper cut-off frequency lower than 30.0 Hz [4,14–16]. Frequencies above 30 Hz were not included to avoid muscle and powerline artifacts [17].
The EEG recordings are analyzed in average reference [17].
Methods
Data from format Brainquick 0, 3 and 4 were imported into MATLAB 2015a (MathWorks, USA). The data was processed by using EEGLAB [18] and the microstates analysis was done using MicrostateAnalysis plug-in created by T. Koenig [19].
We created amplitude maps for each patient separately. Then we created clusters with the help of Atomize and Agglomerate Hierarchical Clustering (AAHC). It is a hierarchical method of clustering where we chose 4 clusters defining them to be our 4 micro-states (for more information about AAHC see [20]). Maps representing microstates are created at one time point, so it is not the average value but the instantaneous amplitude value in the given sample (time point). The microstates analysis use calculation of global field power (GFP) and amplitude topographic maps are only made from samples that match the location of the local maximum GFP curve. The polarity reversals are ignored [2].
Global Field Power
The Global Field Power (GFP) is calculated as the square root of the sum of differences of all the potentials of the individual electrode i, Vi(t), and the mean potential of the electrodes, Vmean(t), at each time, see equation 1. So GFP represents the spatial variance of the EEG signal in each time sample. All GFP points (time samples of the EEG signal) get one GFP curve which represents 19 channels in our case [9].
In equation 1, K is the number of electrodes.
In the GFP curve we detected local maxima. Two vectors of variance values that have mutual offset are compared for that. The local maxima are detected by the derivative—the difference and its direction are exam-ined in three adjacent time points.
Topographic maps and calculated parameters
The distribution of the electrodes corresponds to the standard system 10-20. 19 values (for individual channels) are interpolated by the averaging method to obtain the resulting scalp potential distribution—maps. Resulting maps are classified by AAHC algorithm see [20].
We investigated the differences in three commonly used parameters, being duration, occurrence and contri-bution for each microstate.
The mean duration (lifespan) of a microstate M is the average length of time for which the microstate M remains stable. It is the average time covered by a given microstate class. Duration is the time information about how long the microstate M lasts in unchanged form. [5, 9]
The mean duration is calculated from durations of microstates di and number of microstates NM, see equation 2.
The frequency of occurrence of each microstate M is the mean number of occurrences NM per time t in seconds (1-second window) when the microstate becomes dominant, see equation 3. [5, 9]
The contribution of a microstate M is calculated as the duration of a microstate di from one class in total time of the EEG recording, see equation 4. [5, 9].
Evaluation
In microstates we evaluate the actual distribution of the potential in the map (resolution of four states), the duration of the microstates and the transitions between the microstates. Results were discussed considering feasibility and differences between signals of the epilepsy patients and controls. We computed medians and statistical comparison for both cases and four microstates. Medians represent the middle value of datasets. Statistical comparison was calculated by a two-sample statistical test like in [6] and [21], but we chose independent, nonparametric test Mann-Whitney.
We have chosen a non-parametric test because we compared only 6 epileptic signals and 10 non-epileptic signals and for such a small number of samples we cannot assume normality as in [22].
We have also calculated mean topographic maps for epileptic and non-epileptic datasets for all 4 microstates and a grand mean over all of the recordings.
Results
We computed the mean of the topographic maps of the patients with epilepsy, see Fig. 1, and of the non-epileptic controls, see Fig. 2.
For the comparison of the two groups we calculated a grand mean over all of the subjects in this study, see Fig. 3.
From figures 1–3 we can say that our microstate 1 corresponds with microstate D in the study [8], our microstate 2 corresponds with microstate A, our micro-state 3 corresponds with microstate C and our microstate 4 corresponds with microstate B in the same study.
We computed parameters of the microstates analysis: duration, occurrence and contribution. We computed these parameters for each subject and then we made the median over the two groups—patients with epilepsy, see Table 1, and non-epileptic controls, see Table 2.
The parameter global variance (GEV) explained the percentage of the explained information by each micro-state across participants. The global variance for the epileptic patients was 69.78%. The global variance for the non-epileptic controls was 73.81%.
We used the non-parametric Mann-Whitney test of hypothesis between patients with epilepsy and non-epileptic control datasets. The p-values of this tests are shown in Table 3.
There is a significant difference in microstate 1 in the occurrence and in the contribution at significance level 0.01. In the microstate 2 there is a significant difference between the two groups in the duration at significance level 0.01 and in the occurrence and the contribution at significance level 0.05. In the microstate 3 there is a significant difference in the occurrence at significance level 0.01. There is a significant difference in microstate 4 in the duration and the contribution at significance level 0.01.
Discussion
We compared the EEG recordings of two groups: the patients with epilepsy and the non-epileptic controls (meaning the EEG recordings with no epileptic pathology according to the expert). We evaluated these recordings by microstates parameters duration, occur-rence and contribution. The main finding of our research is that the distribution of test file maps corresponds to each of the 4 classes (microstates A, B, C and D) from available literature. We have found that the microstates dynamic is different between controls and patients with epilepsy.
Our results of the two-tailed non-parametric Mann-Whitney test of hypothesis in Table 3 show that there is a significant difference between the two groups in following particular parameters in particular micro-states. The duration parameter is significantly different with a significance level of 0.01 between both groups in microstates 2 and 4. For microstate 3 no significant difference was proven. We assume that these results for microstates 2 and 4 confirm our hypothesis that a lower number of the spatial variability changes and hence a longer duration of the microstates. The reason for this physiological hypothesis is that epileptic seizures are accompanied by high synchronization of the neurons [23]. For this reason, we assume that microstates 2 and 4 represent higher synchronization of the epileptic seizures. The occurrence parameter is significantly different with a significance level of 0.01 between the two groups in microstates 1 and 3, and with a signifi-cance level of 0.05 for microstate 2, so it can be also used for differentiation. As the occurrence parameter reflects the mean value of number of times the microstate occurs per second, our results show that the microstates 1–3 alternate more for the control subjects. The smaller difference of the occurrence parameter between epileptic and non-epileptic subjects for micro-states 2 and 4 could be due to a large difference in the duration parameter. The occurrence of the micro-states could be suppressed for a higher duration. The contribution parameter is significantly different with a significance level of 0.01 between the two groups in microstates 1 and 4. On the significance level 0.05 could differ the microstate 2 to for the contribution parameter. It seems that the occurrence for microstate 1 is of a higher value for control subjects, meaning more alternations of this microstate. Also, the contribution parameter seems to be higher for microstate 1 compared to the epileptic group, meaning more power in this microstate. The occurrence of microstate 1 for the epileptic group may be suppressed due to an epileptic seizure.
The duration parameter has lower values for subjects with some mental or neurological disorders [24]. In our study the duration parameter was higher in subjects with epilepsy for microstates 1–3. These values could be caused by neurons that synchronize during epileptic induction, creating high polarity wavelengths and having longer activity. The typical range of the micro-state duration is between 60 and 120 ms on average [2]. On the other hand, there are studies that reported lower values, for example in the studies [21, 25] is the mean duration between 36.87 and 81.72 ms. In our study we found surprisingly low values of microstate B. This can be caused by the artifact’s residuals manifestation in these topographic maps.
The microstate 2 is proven to be distinctive for all the parameters at significance level 0.05 (in the worst case). On the other hand, microstate 3 is distinctive only for the occurrence parameter. Assuming this hypothesis, the microstates of epileptic patients in Table 1 seem to have a longer duration and seem to have lower occurrence and alternate with smaller frequency compared to control subjects. The investigated patients had at least one epileptic seizure during the measurement. The epileptic seizure is also manifested by increased amplitude (epi-leptic spike) when the activity is manifested through multiple channels. So it is possible that the difference in the duration or occurrence of microstates is affected by an epileptic seizure.
We have used the band-pass filter with cut-off fre-quencies 0.5 and 30.0 Hz. This type of filtering was used also in studies like [14, 15, 26]. The band-pass filtering with the shorter range 2–20 Hz was used in [27], with the wider range 1–50 Hz was used in [16]. In our review we did not find a publication that would deal with the influence of filtering on the final microstates maps. Part of the artifacts, like higher muscle artifacts and line noise artifact, should be supposed by the band-pass filtering. We did not use any other filtering to avoid artifacts. In some studies, like in [28], the method of independent component analysis is used for removing the slow artifacts caused by the eye movement. In some other studies they used only the band-pass filtering [4]. We have chosen only the band-pass filtering, so our results could be therefore compared with studies that do not use any further suppression of the artifacts.
In our study, we used average montage even with the knowledge of its limitations in the form of susceptibility to the distribution of some artifacts [29]. However, average montage errors are not so high compared to other montages [30, 31]. And most studies use just average montage, for example studies [14, 24, 32]. For this reason, it is possible to compare the results of microstate studies among themselves.
Further research with a larger number of subjects or with different types of epileptic activity is needed to continue with this feasibility study. A larger dataset can allow us to evaluate the effect of artifacts on the resulting microstates. It is necessary to verify the effect of neuronal synchronization by an intra-individual comparison of ictal and interictal recordings and by an inter-individual resting state in the recordings of epilep-tic and non-epileptic patients.
Conclusion
We analyzed EEG recordings by calculating the GFP curves to extract the microstates and obtain the ampli-tude topographic maps of these microstates. We classified the resulting topographical maps with AAHC algorithm to show the feasibility of microstate analysis in EEG recordings with epileptic activity. EEG record-ings were labeled by an expert as epileptic and non-epileptic. We obtained four microstates in epileptic EEG recordings and we assume that they correspond to the microstates A–D stated in [8], where the microstates were used on resting-state EEG. So we have found out that analyzing EEG recordings with epileptic activity using microstates analysis is feasible since these record-ings have similar spatial maps as in other studies.
Conflict of interest declaration
The authors declare that there is no conflict of interest regarding the publication of this article.
Statement of informed consent
The study protocol and patient informed consent have been approved by the Hospital Na Bulovce ethical com-mittee.
Protection of human subjects
The procedures followed were in compliance with the ethical standards of the responsible committee on human experimentation (institutional and national) and with the World Medical Association Declaration of Helsinki on Ethical Principles for Medical Research Involving Human Subjects.
Acknowledgement
This work was supported by the Grant Agency of the Czech Technical University in Prague with the topic: The topographic brain mapping, grant no. SGS18/158/
OHK4/2T/17; Feature space analysis using linear and non-linear reduction of EEG space dimensions, grant no. SGS18/159/OHK4/2T/17; and by the Grant Agency of the Czech Republic with the topic: Temporal context in analysis of long-term non-stationary multidimensional signal, register no. 17-20480S.
We thank Svojmil Petranek, MD and Hospital Na Bulovce, Department of Neurology, Prague, Czech Republic.
Ing. Václava Piorecká
Department of Biomedical Technology
Faculty of Biomedical Engineering
Czech Technical University in Prague
nám. Sítná 3105, CZ-272 01 Kladno
E-mail: vaclava.piorecka@fbmi.cvut.cz
Phone: +420 224 357 996
Zdroje
-
Lehmann, D., Faber, P. L., Galderisi, S., Herrmann, W. M., Kinoshita, T., Koukkou, M., Mucci, A., Pascual-Marqui, R. D., Saito, N., Wackermann, J., Winterer, G., Koenig, T.: EEG microstate duration and syntax in acute, medication-naïve, first-episode schizophrenia: a multi-center study. Psychiatry Research: Neuroimaging 138(2), 141–156 (2005). DOI: 10.1016/j.pscychresns.2004.05.007.
-
Michel, C. M., Koenig, T.: EEG microstates as a tool for studying the temporal dynamics of whole-brain neuronal networks: A review. NeuroImage 180(Pt B), 577-593 (2018). DOI: 10.1016/j.neuroimage.2017.11.062, ISSN 10538119.
-
Britz, J., Van de Ville, D., Michel, C. M.: BOLD correlates of EEG topography reveal rapid resting-state network dynamics. NeuroImage 52(4), 1162–1170 (2010). DOI: 10.1016/j.neuro-image.2010.02.052.
-
Koenig, T., Prichep, L., Lehmann, D., Sosa, P. V., Braeker, E., Kleinlogel, H., Isenhart, R., John, E.: Millisecond by millisecond, year by year: Normative EEG microstates and developmental stages. NeuroImage 16(1), 41–48 (2002). DOI: 10.1006/nimg.
2002.1070. -
Milz, P., Faber, P., Lehmann, D., Koenig, T., Kochi, K., Pascual-Marqui, R.: The functional significance of EEG microstates – associations with modalities of thinking. NeuroImage 125, 643–656 (2016). DOI: 10.1016/j.neuroimage.2015.08.023.
-
Kikuchi, M., Koenig, T., Wada, Y., Higashima, M., Koshino, Y., Strik, W., Dierks, T.: Native EEG and treatment effects in neuroleptic-naive schizophrenic patients: Time and frequency domain approaches. Schizophrenia Research 97(1-3), 163–172 (2007). DOI: 10.1016/j.schres.2007.07.012.
-
Nishida, K., Morishima, Y., Yoshimura, M., Isotani, T., Irisawa, S., Jann, K., Dierks, T., Strik, W., Kinoshita, T., Koenig, T.: EEG microstates associated with salience and frontoparietal networks in frontotemporal dementia, schizophrenia and Alzheimer’s disease. Clinical Neurophysiology 124(6), 1106–1114 (2013). DOI: 10.1016/j.clinph.2013.01.005.
-
Kikuchi, M., Koenig, T., Munesue, T., Hanaoka, A., Strik, W., Dierks, T., Koshino, Y., Minabe, Y., Yoshikawa, T.: EEG microstate analysis in drug-naive patients with panic disorder. PLoS ONE 6(7), e22912 (2011). DOI: 10.1371/journal.pone.
0022912. -
Khanna, A., Pascual-Leone, A., Michel, C. M., Farzan, F.: Microstates in resting – state EEG: Current status and future directions. Neuroscience & Biobehavioral Reviews 49, 105–113 (2015). DOI: 10.1016/j.neubiorev.2014.12.010.
-
Hamandi, K., Routley, B. C., Koelewijn, L., Singh, K. D.: Noninvasive brain mapping in epilepsy: Applications from magnetoencephalography. Journal of Neuroscience Methods 260, 283–291 (2016). DOI: 10.1016/j.jneumeth.2015.11.012.
-
Arab, M. R., Suratgar, A. A., Ashtiani, A. R.: Electroencephalo-gram signals processing for topographic brain mapping and epilepsies classification. Computers in Biology and Medicine 40(9), 733–739 (2010). DOI: 10.1016/j.compbiomed.2010.06.
001. -
Formaggio, E., Storti, S. F., Bertoldo, A., Manganotti, P., Fiaschi, A., Toffolo, G. M.: Integrating EEG and fMRI in epilepsy. NeuroImage 54(4), 2719–2731 (2011). DOI: 10.1016/j.
neuroimage.2010.11.038. -
Marušič, P.: Chyby při hodnocení a interpretaci EEG (oral presentation). 32. Slovenský a český neurologický zjazd, 28. 11. – 1. 12. 2018. Martin, Slovak Republic, [presented No-vember 28th 2018, Section: Epilepsia 2], www.scnz2018.sk.
-
Santarnecchi, E., Khanna, A. R., Musaeus, C. S., et al.: EEG Microstate Correlates of Fluid Intelligence and Response to Cognitive Training. Brain Topography 30(4), 502–520 (2017). ISSN 0896-0267. DOI: 10.1007/s10548-017-0565-z.
-
Koenig, T., Lehmann, D., Merlo M. C. G., Kochi, K., Hell, D., Koukkou, M.: A deviant EEG brain microstate in acute, neuroleptic-naive schizophrenics at rest. European Archives of Psychiatry and Clinical Neuroscience 249(4), 205–211 (1999). DOI: 10.1007/s004060050088. ISSN 0940-1334.
-
Khanna, A., Pascual-Leone, A., Farzan, F.: Reliability of resting-state microstate features in electroencephalography. PLoS One 9(12), e114163 (2014). DOI: 10.1371/journal.pone.0114163.
-
Siclari, F., Baird, B., Perogamvros, L., et al.: The neural correlates of dreaming. Nature Neuroscience 20(6), 872–878 (2017). DOI: 10.1038/nn.4545. ISSN 1097-6256.
-
Delorme, A., Makeig, S.: EEGLAB: An open source toolbox for analysis of single-trial EEG dynamics including independent component analysis. Journal of Neuroscience Methods 134(1), 9–21 (2004). DOI: 10.1016/j.jneumeth.2003.10.009.
-
Koenig, T.: The EEGLAB plugin for microstates (2017), Available from: http://www.thomaskoenig.ch/index.php/
software/microstates-in-eeglab/ -
Rokach, L., Maimon, O.: Clustering methods. In: Maimon O., Rokach L. (eds). Data Mining and Knowledge Discovery Handbook. Springer US, Boston, MA, 321–352 (2005). DOI: 10.1007/0-387-25465-X_15.
-
Liu, W., Liu, X., Dai, R., Tang, X.: Exploring differences between left and right hand motor imagery via spatio-temporal EEG microstate. Computer Assisted Surgery 22(sup1), 258–266 (2017). DOI: 10.1080/24699322.2017.1389404.
-
Ihl, R., Dierks, T., Froelich, L., Martin, E. M., Maurer, K.: Segmentation of the spontaneous EEG in dementia of the Alzheimer type. Neuropsychobiology 27(4), 231–236 (2004). DOI: 10.1159/000118986.
-
Paulus, M., Komarek, V., Prochazka, T., Hrncir, V., Sterbova, K.: Synchronization and information flow in EEGs of epileptic patients. IEEE Engineering in Medicine and Biology Society 20(5), 65–71 (2001). DOI: 10.1109/51.956821.
-
Gao, F., Jia, H., Wu, X., Yu, D., Feng, Y.: Altered resting-state EEG microstate parameters and enhanced spatial complexity in male adolescent patients with mild spastic diplegia. Brain Topography 30(2), 233–244 (2017). ISSN 0896-0267. DOI: 10.1007/s10548-016-0520-4.
-
Seitzman, B. A., Abell, M., Bartley, S. C., Erickson, M. A., Bolbecker, A. R., Hetrick, W. P.: Cognitive manipulation of brain electric microstates. NeuroImage, 146, 533–543 (2017). DOI: 10.1016/j.neuroimage.2016.10.002.
-
von Wegner, F., Knaut, P., Laufs, H.: EEG microstate sequences from different clustering algorithms are information-theoreti-cally invariant. Frontiers in Computational Neuroscience, 12–70 (2018). ISSN 1662-5188. DOI: 10.3389/fncom.2018.00070.
-
Miltz, P., Pascaul-Marqui, R. D., Achermann, P., Kochi K., Faber, P. L.: The EEG microstate topography is predominantly determined by intracortical sources in the alpha band. NeuroImage. 162, 353–361 (2017). DOI: 10.1016/j.neuroimage.
2017.08.058. -
Dinov, M., Leech R.: Modeling uncertainties in EEG micro-states: Analysis of real and imagined motor movements using probabilistic clustering-driven training of probabilistic neural networks. Front Hum. Neurosci, 11–534 (2017). DOI:10.3389/
fnhum.2017.00534. -
Dien, J.: Issues in the application of the average reference: Review, critiques, and recommendations. Behavior Research Methods. Instruments. & Computers, 30(1). 34–43, (1998).
-
Lei, X., Liao, K.: Understanding the influences of EEG reference: A large-scale brain network perspective. Front Neurosci. 11(205), (2017). DOI: 10.3389/fnins.2017.00205.
-
Hagemann, D., Naumann, E., Thayer, J. F.: The quest for the EEG reference revisited: A glance from brain asymmetry research. Psychophysiology, 38(5), 847–857, (2001). Cam-bridge University Press. DOI: 10.1111/1469-8986.3850847.
-
Gao, F., Jia, H., Wu, X., Yu, D., Feng, Y.: Altered resting-state EEG microstate parameters and enhanced spatial complexity in male adolescent patients with mild spastic diplegia. Brain Topography 30(2), 233–244 (2017). ISSN 0896-0267. DOI: 10.1007/s10548-016-0520-4.
Štítky
BiomedicínaČlánek vyšel v časopise
Lékař a technika
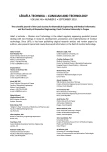
2018 Číslo 3
Nejčtenější v tomto čísle
- EEG MICROSTATES ANALYSIS IN PATIENTS WITH EPILEPSY
- EFFECT OF SAMPLING RATE ON THE ACCURACY OF MEASUREMENT OF NEONATAL OXYGEN SATURATION EXPOSURE
- BIPHASIC CALCIUM PHOSPHATE SCAFFOLDS DERIVED FROM HYDROTHERMALLY SYNTHESIZED POWDERS
- FLEXIBLE TEG ON THE ANKLE FOR MEASURING THE POWER GENERATED WHILE PERFORMING ACTIVITIES OF DAILY LIVING